Big Data Analytics: The Next Evolution In Drug Development
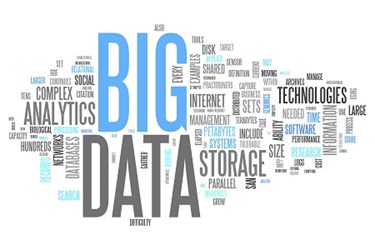
By Deb Phippard, PhD, VP, research, Precision for Medicine and Jared Kohler, PhD, managing director, analytics, Precision for Medicine
The drug development process is complex and financially risky. A recent study by The Tufts Center for the Study of Drug Development estimates the cost of developing a new drug at $2.6 billion and suggests that costs of drug development are rising with a compound annual growth rate of 8.5 percent. These rising costs are largely driven by increases in out-of-pocket costs, such as larger clinical trial sizes and higher failure rates for drugs required to demonstrate superiority. Even when considering the efficacy of the 10 highest-grossing approved drugs in the United States, the fact is that for every patient a drug does help, between three and 24 patients fail to show improvement after treatment. Clinicians are typically forced to address this variability in patient outcomes with a trial-and-error approach to intervention, increasing healthcare costs and adding a burden to the patient.
Drug Developers, Providers, And Patients Need A Better Option
High-throughput technologies are ushering in the era of Big Data in drug development, allowing researchers to assay patients in terms of their genome, epigenome, proteome, metabolome, and microbiome. Precision medicine initiatives are being undertaken to tailor disease treatment by taking into account individual variability in molecular and cellular systems. A biomarker- and technology-driven approach to developing targeted therapies and patient selection strategies has the potential to increase success in the drug development process, decrease cost, and ultimately improve patient outcomes with directed intervention.
Next-generation sequencing (NGS) has already made the shift from academia to industry and the clinic. Barriers are being removed and costs continue to decrease for data generation, storage, and computing; however, we are still faced with terabytes of data to analyze and interpret. Traditional statistics are not equipped to handle these Big Data sets due to the problems resulting from dimensionality and correlation inherent in genetic data. Scientists need advanced tools specifically engineered to meet modern research demands. Fortunately, biopharma can benefit from the large investment made by organizations in other areas, such as natural language processing and business intelligence. This has resulted in a boom in cutting-edge algorithms that are being repurposed for use in precision medicine. In this era of Big Data and technology, the future of drug development will be shaped by flexible frameworks designed to discover complex signals through the merger of predictive analytics and systems biology.
Big Data Analytics Has Advanced Beyond The Hype
Data modeling, data mining, and machine learning will be integral to making sense of NGS data and enabling a data-driven drug development process. Implementing tools that leverage the strength of multiple algorithms or combining tools that use different techniques is recommended. It will also be critical to ensure algorithms are considerate of the complex structure in biological data and capable of incorporating biological knowledge.
Machine learning, which is a class of techniques enabling computers to recognize and learn patterns in data for use in future predictions, will be at the forefront of Big Data analytics due to its ability to solve the types of complex problems inherent in human biology and drug response. Supervised machine learning approaches that use sophisticated mathematical algorithms to optimize complex predictor functions on a predefined outcome can be used to reduce Big Data to a more manageable set of biomarker candidates, or “features.” Tools that take an ensemble approach may lead to discovery of different types of effects, which is a worthy consideration when attempting to understand human biology.
Machine learning techniques are particularly desirable in genomics, as smart algorithms can be designed to handle complex biological structures as well as knowledge about biological systems. NGS assays have the potential to produce millions of data points ranging from single-point mutations to gene expression levels to methylation status. Molecular and cellular biological systems are complex and comprise networks, pathways, and structure. Machine learning techniques have improved ability over traditional statistics to discover the complex signals underlying human biological response to drugs through collectively evaluating this system by naturally grouping variables and extracting patterns according to biologically relevant units of variations, signaling pathways, or gene regulatory networks.
Helping Researchers Make Sense Of Big Data
Big Data has the potential to enable a precision medicine-focused drug development process, resulting in smaller, shorter clinical trials and, ultimately, increased benefit-risk profiles for patients with a particular biomarker profile. To realize this, it is critical that researchers get the support necessary to make sense of Big Data being generated in NGS pipelines. Life science companies with precision medicine initiatives are addressing their NGS analysis needs by building specialty groups for bioinformatics and advanced analytics, partnering with thought leaders, and adopting technology-based solutions for their NGS pipelines. Entrepreneurial organizations and vendors are responding by developing software and cloud-based solutions powered by predictive analytic engines and user-friendly graphical interfaces. The future of precision medicine is bright as the smart use of Big Data, analytics, and technology will bring much needed relief from increasing drug development costs.